How AI is changing retail
By Kathryn Casna
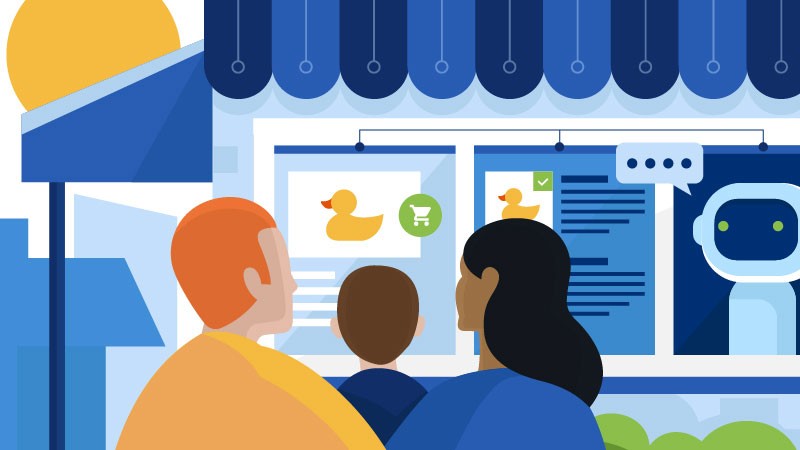
Like many industries, retail is transforming thanks to artificial intelligence (AI). According to NVIDIA’s State of AI in Retail and CPG report, more than 60% of retail respondents planned to increase their AI infrastructure. Artificial intelligence in retail crunches data, automates processes, and can improve the customer experience — and your competitors may already be using this technology. Grand View Research expects the global smart retail market — which combines AI, virtual reality, augmented reality, and the Internet of Things — to grow at a rate of 29.1% by 2030. Ever-higher customer expectations and the need to use the retail workforce more efficiently drives the growth of AI in online and brick-and-mortar retail.
Retailers use AI in an effort to improve customer satisfaction, lower labour costs, and boost net revenue. But understanding emerging technology and how it can best serve your business can be a challenge. Read on for a primer on AI and to learn ways you can leverage the benefits of AI in retail.
What is artificial intelligence?
AI is a computer or program that has been taught to mimic human skills or behaviours, often to reduce the need for a human to perform a given task. The idea of AI began as early as the 1950s, but it took decades of research, the flourishing of the Internet, and computers with modern processing power to develop the multiple kinds of AI that exist today.
- 1950: Alan Turing develops a test to gauge computer intelligence.
- 1955: Scientists invent the first AI program, Logic Theorists.
- 1963 The U.S. Defense Advanced Research Projects Agency (DARPA) funds AI at the Massachusetts Institute of Technology.
- 1986: Carnegie Melon builds the first autonomous car.
- 1997: Deep Blue becomes the first computer to beat a chess champion, Gary Kasparov.
- 1997: Dragon Systems builds the first publicly available speech-recognition software.
- 2002: The University of Alberta launches Alberta Innovates Centre for Machine Learning (AICML), which will later become Amii, in an effort to attract the best AI researchers in the world.
- 2007: Amii’s AI program, Chinook, is the first to officially solve the game of checkers when it beats Marion Tinsley, a human champion.
- 2011: The Google Brain project launches with the goal of improving search results using AI.
- 2012: University of Toronto researchers develop AlexNet, a significant milestone in computer vision.
- 2015: OpenAI, the creator of the now famous ChatGPT, forms.
- 2017: Canada launches NextAI, an AI innovation hub for entrepreneurs and researchers.
- 2022: OpenAI releases ChatGPT3 to the public, launching AI into everyday use.
- 2023: Microsoft releases Copilot (formerly Bing), and Google releases Gemini (formerly Bard)
Sources :
- The History of Artificial Intelligence (2017, August 28). Science in the News Harvard.
- A Brief History of AI in Canada. Canada.ai
- Google Brain: The Brains Behind Your Search Engine (2021, June 3). History of Data Science.
- OPENAI, INC. OpenCorporates.
- ChatGPT — Release Notes. OpenAI Help Center.
- Announcing Microsoft Copilot, your everyday AI companion (2023, September 21). Official Microsoft Blog.
- Introducing Gemini: our largest and most capable AI model (2023, December 6). The Keyword.
Examples of AI tools
Many kinds of AI tools are available; here are the ones retailers often use for their businesses.
Machine learning
In this subset of AI, programmers develop algorithms (or automated instructions) that enable a computer to learn new topics or skills without anyone explicitly programming it, usually with the goal of the computer behaving more like a human would. A conversational AI program may learn from recorded tech-support conversations, while a generative AI program may study images of your products.
Deep learning
These models, which are programs that apply algorithms, help turn mountains of data into useful insights — all without having to hire expensive data analysts or go down a spreadsheet rabbit hole. This tech often pairs with predictive analytics.
Predictive analytics
This field of data analysis leverages data, statistics, and modelling to help extrapolate how your business will perform — and helps you make decisions to maximize your performance. One decision predictive analytics may guide, for example, is which items to discount and when, based on how quickly an item flies off the shelves. The models you’ll leverage depend on your industry and needs.
Computer vision
This technology uses smart cameras to allow the AI to “see” the world around it, allowing retailers to track their physical and virtual shelves 24/7. With the collected visual data, an AI can power self-checkout, manage inventory, or model customer behaviour.
Conversational AI
This type of AI system uses natural language processing (NLP) so customers can have human-like conversations with chatbots, where they can ask for product information or troubleshoot technical problems. It helps service and support staff by automating some communication with customers. This category includes autocorrect and AI transcription tools as well.
Generative AI
This type of AI generates new content, including text, images, or other types of data. It helps retail businesses create digital assets and inspire ideas. You may use it to generate graphics, create a blog post, or write code for an app.
Machine learning
A branch of AI that enables computers to study structured and annotated data (or data that’s been labelled to help AI understand it more), then use statistics to categorize information, predict what will happen next, and find connections
Deep learning
A more intense kind of machine learning that can perform analytical or physical tasks much like the human brain
Predictive analytics
A field of data analytics that uses machine learning to analyze historical data in order to determine future performance
Computer vision
A type of AI that studies large quantities of images by breaking them down pixel by pixel so it can recognize things like people, products, and shopping bags
Conversational AI
An AI system that studies samples of human language, analyzes them, and creates an appropriate human-like response
Generative AI
A type of AI that digests large amounts of data, then creates something that’s similar but a little bit different — mimicking a collage
Sources:
How AI is used in retail
AI in the retail industry is typically used in an effort to streamline workflows, save on labour costs, market more effectively, and improve security. Among both online and brick-and-mortar stores, you could use it to streamline the processes we cover below.
Inventory management and demand forecasting
Computer vision can keep an eye on your shelves and storage areas to help you track inventory more efficiently. It can also use deep learning and predictive analysis to combine your inventory data over time with information about competitors and customer sentiment to help predict demand. Some systems will even automatically order products when they’re low.
Customer engagement
Both online and brick-and-mortar retailers can leverage AI to boost customer engagement. In this case, AI uses computer vision and transactional data to study customer behaviour, taking particular note of when customers purchase multiple items. Many systems even consider customer demographics. These AIs use deep learning to analyze the kinds of products customers buy and predictive analytics to recommend those items to similar customers.
Online, this technology can provide personalized product recommendations. In stores, it has the potential to enable digital shelf signage that recommends related items as customers walk by. If customers can use these displays to ask questions or get product specs, AI can boost engagement even more.
Customer support
AI-powered chatbots can help customers track orders, initiate a return, troubleshoot technical issues, answer common questions, collect feedback and more. And as mentioned earlier, automated support can free up your customer service reps to focus on more complex issues.
Payment processing
Since the COVID-19 pandemic, two thirds of adults worldwide now make or receive digital payments. While maintaining compliance with data privacy regulations, businesses have a huge opportunity to collect and leverage data from purchases in order to meet rising security, efficiency, and customer satisfaction challenges.
With all this data, deep learning AIs can find anomalies and flag possible fraudulent activity. Meanwhile, AIs using predictive analytics can predict future payment volume so you can plan accordingly. And for businesses that process a lot of returns or refunds, conversational AI can automate customer service to support these transactions.
The future of AI in retail
While many retailers already use AI, this technology continues to evolve. The more data retailers produce and collect about transactions, customer behaviour, and their products, the more AI can do.
- From 2022 to 2023, Canada budgeted $2.57 billion for external AI research, funding, and development.
- Canadian FinTech (which includes some AI technologies used in retail) grew 30% from 2018 to 2021.
- More than 60% of retailers plan to increase their AI infrastructure in the next 18 months.
- Canada ranks fifth out of 62 countries for AI capacity (a measure of AI scale and intensity).
- Just 2.2% of Canadian retail companies use natural language recognition and 1.1% use face, image, or pattern recognition in their businesses.
Sources:
- Impact and opportunities: Canada's AI ecosystem – 2023. Deloitte (PDF).
- FinTech investment in Canada is on track to nearly double in 2022 (2022, April 23). Fintech Global.
- State of AI in Retail and CPG. Nvidia.
- Applications related to artificial intelligence technologies, by industry and enterprise size (2023, July 28). Statistics Canada.
AI and marketing
As AI tools collect more customer data, you can use them to more effectively boost your marketing ROI. AI can crunch customer data down to the individual level, allowing you to automate more personalized deals, offers, and incentives. Meanwhile, predictive AI can study data from past campaign performance and pair the data with current trends to help you decide what kinds of campaigns to invest in.
Personalized shopping
As more customers shop online, AI can learn more about their preferences and demographics to create automated, personalized digital shopping experiences. For repeat visitors, you can place previously viewed products or location-based offers on a personalized homepage. For new visitors, you can share your company’s vision, your best customer reviews, and other trust-building content.
AI-powered checkout
As computer vision improves, brick-and-mortar stores can safely provide self-checkout options with less risk of theft. In some pioneering stores, customers skip the checkout process altogether. Cameras capture the customers selecting items from shelves while AI identifies them, tallies the bill, and automatically charges the customer’s preferred payment method. AI processing could also provide additional payment options like eye recognition.
Product placement
Many retailers have in-store cameras to prevent theft, but the footage they generate could be leveraged by AI to help business owners optimize store layouts and decide where to place their products. An AI can generate a heatmap of where customers spend the most time, allowing you to place high-margin products in more strategic places and move low-margin products elsewhere.
Prepare for the future of retail
Although AI has been in the works for nearly 75 years, its use in retail is just starting to pick up momentum. Retailers are already using it for personalized marketing, theft and fraud prevention, store and warehouse design, and more. To learn more about AI in retail and discover omnichannel strategies for retailers, check out these other resources.